Rules of the Chemical-Microbial Network
Research Theme Summary:
C-CoMP is working to determine the rules of the chemical-microbe network that regulate the massive transfer of carbon between global reservoirs.
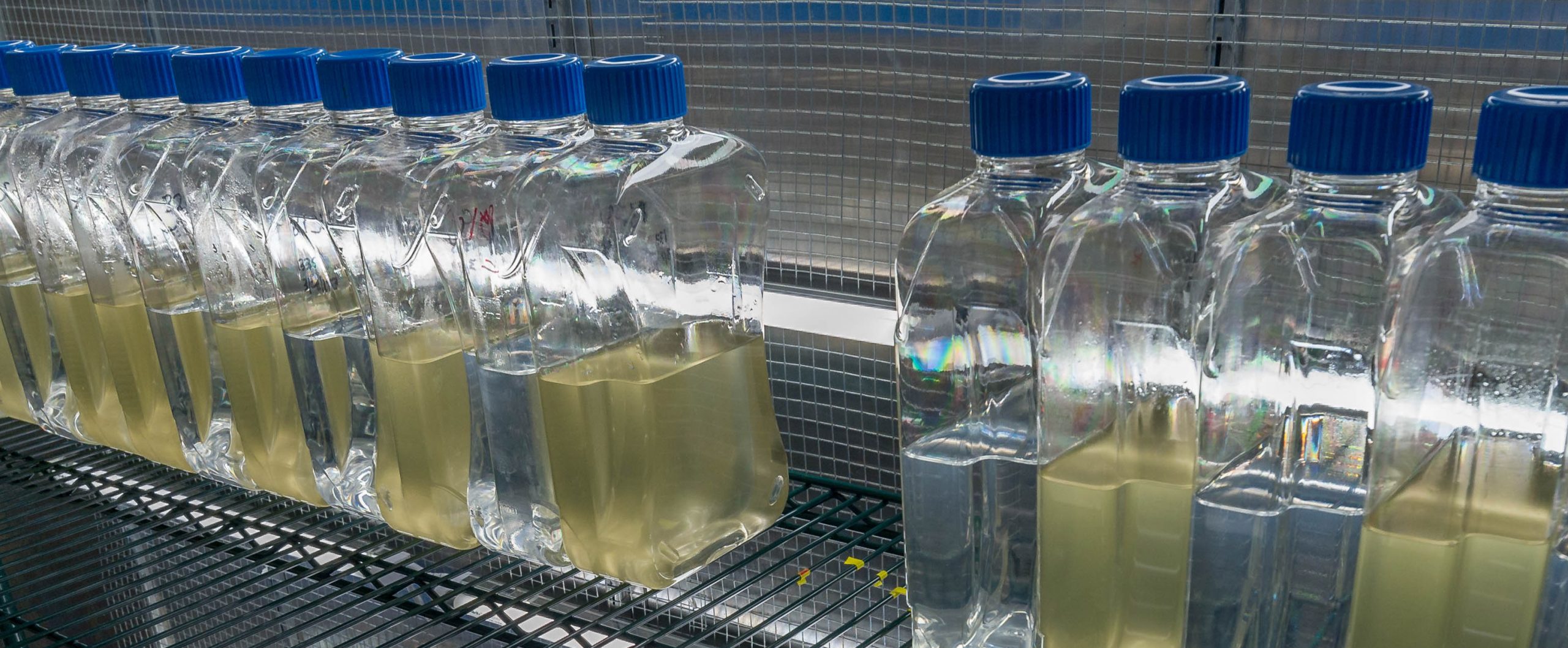
Model systems
We are using laboratory model systems to build and validate conceptual and computational models that capture emergent properties of the chemical-microbe network. Experimental systems that span ranges in throughput, scale, homogeneity, and microbial and viral components are leveraged to build quantitative insights into molecule flux and the factors that control them. Model systems are analyzed using tools in ‘omics (genomics, transcriptomics, proteomics, metabolomics), isotope labeling, and genetic manipulations of microbial phenotypes. We are addressing relationships among chemical features (functional groups, heteroatoms, element stoichiometry) and biological reactivity, microbial uptake and release rates, microbial growth versus efficiency trade-offs, and viral rewiring of metabolism to identify predictable rules of the network. Experiments focusing on representative autotrophic and heterotrophic taxa are the focus here, and link directly to cell- and community-scale modeling.
Field observations (BATS, MVCO)
C-CoMP is leveraging existing partnerships with two time-series stations in the Atlantic Ocean, the open-ocean Bermuda Atlantic Time Series Study (BATS; established in 1988) and the coastal Martha’s Vineyard Coastal Observatory (MVCO; established in 2000) to complement and place findings from model system research into an environmental context. BATS and MVCO have strong annual cycles in ocean physics, nutrient regimes, and microbial communities within which to situate field experiments. Each program has the benefit of ongoing multi-disciplinary data collection through core programs and collaborative research by affiliated investigators, including C-CoMP scientists. Accessibility from Bermuda (BATS) and Woods Hole (MVCO) make these ideal partners for field research, and they offer platforms for comparative analyses to other well- studied ocean systems (e.g., San Pedro Ocean Time series (SPOT), Hawaii Ocean Time-series (HOT)).
Standardized methods are being used to develop and test hypotheses about chemical-microbe interactions and identify molecules and microbes that best predict features of carbon flux. We are planning to use autonomous oceanographic instruments to measure concentrations and fluxes of target bioreactive molecules at the necessary spatial and temporal coverage and to collect targeted surface ocean samples for ‘omics research. Undergraduates in capstone experiences and Bridge to PhD (B2P) Fellows will be involved in method testing for MVCO field surveys and two C-CoMP cruises.
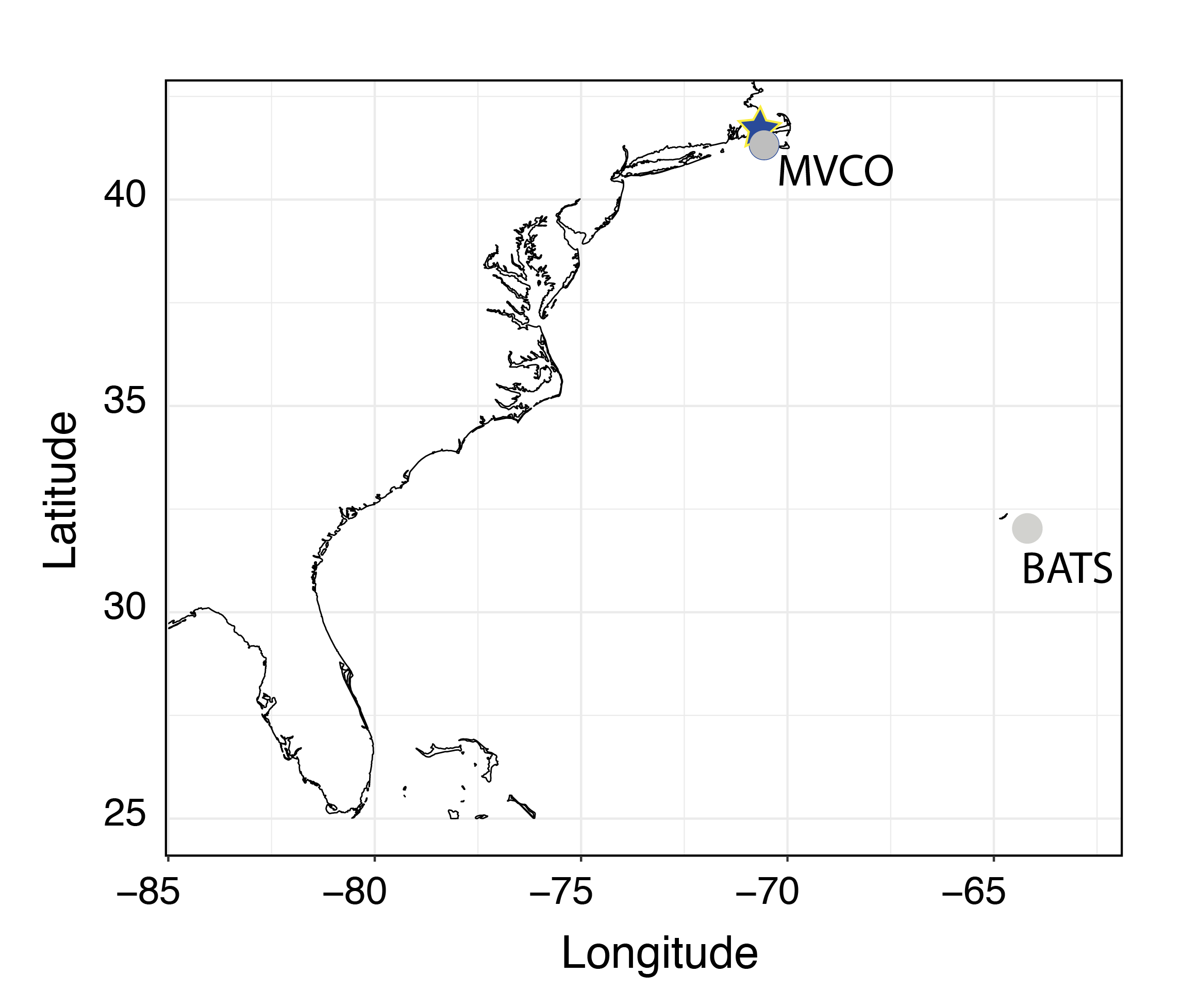
Chemical-microbe network models
C-CoMP is working to develop data-driven models and mechanistic models. Data-driven models will scale and integrate heterogeneous data (from new and existing synthetic and natural marine microbial communities) to search for patterns, dependencies, and inconsistencies that offer insights into the roles of microbial metabolites. The models will consist of networks of correlations in abundance, occurrence, and other metrics of relatedness connecting microbes, microbial genes, and metabolites. This framework will be enhanced with machine learning algorithms to discern microbe-microbe and microbe-metabolite interaction patterns to generate and test hypotheses about underlying principles.
Mechanistic models translate ‘omics information and environmental boundary conditions into quantitative predictions of expected phenotypes. These models can be compared with observations to enable assessment of how metabolite composition and exchange shape communities. To facilitate these comparisons, we are initially focusing on representative autotrophic and heterotrophic taxa for which genomic data and genetic and phage tools are available, and for which physiological constraints will be investigated in model organism and field studies. We are using genome-scale reconstructions of the metabolic networks of these microbes to link intracellular circuits with influx/outflux of metabolites across cell boundaries, as the basis for implementing flux balance analysis (FBA) models.
FBA models are being used in 3 different modalities:
- First, standard biomass optimizations used to predict growth rates and metabolite uptake/ secretion are being validated using measurements from model system experiments, field studies, and data-driven models.
- Second, recently developed algorithms for inverse FBA (invFBA) will test implementation of both standard biomass optimization and more complex metabolic “goals” as drivers of network function. Pathways leading to the largest gains in quality of the fit to experimental data will suggest rules governing cell excretion of dissolved organic carbon.
- Third, “dynamic FBA” (dFBA) will be used to include exchanges between microbial cells, explicitly modeling the dynamics of multi-species communities and predicting interactions arising from competition or metabolite exchange. Our COMETS (Computation of Microbial Ecosystems in Time and Space) dFBA platform enables community simulations with spatial resolution (e.g., taking into account light and nutrient gradients) in which cells communicate via diffusion and local experience rather than bulk conditions.
Knowledge Transfer
This research advances the following Knowledge Transfer aims:
- Ocean data ingestion - data-driven exploration of chemical-microbe co-occurrence patterns
- Software development - visualization of multi-‘omics model system and field data
- Molecular biology - experimentally testing predicted chemical-microbe linkages
- Technology - collecting targeted field samples for hypothesis testing using AUV technology and chemical instrumentation